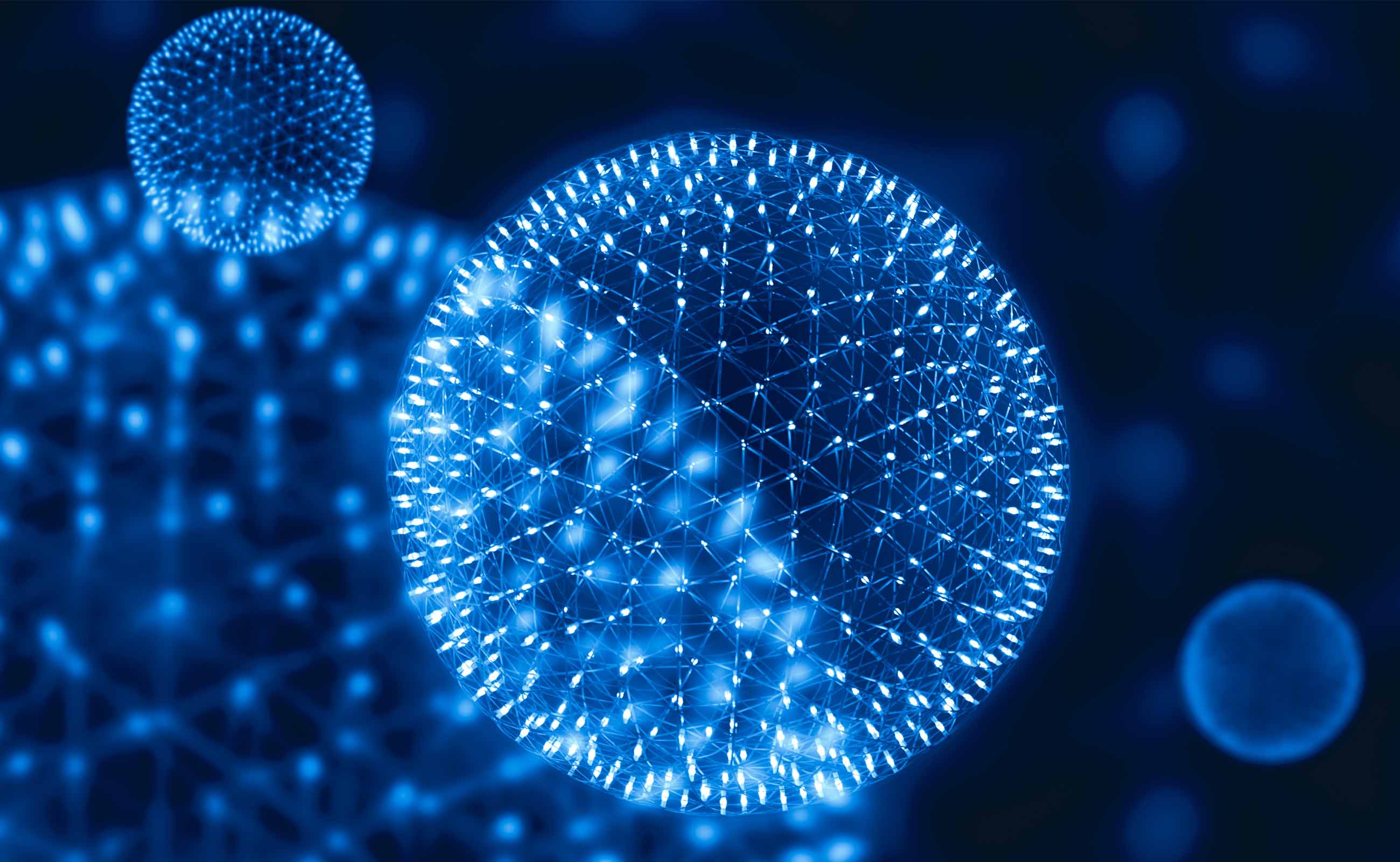
Artificial intelligence is changing how scientific discoveries are made, and here in ESCAPE it’s a big part of our work to make scientific knowledge easier to create and reuse.
Have you seen our Sixty Second Adventures in Artificial Intelligence? If so, and you’d like to know more, try these:
If you’re comfortable with technical details, then the rest of this page will give you some useful resources for our work in astronomy and physics, but keep in mind that not all of this will be easily accessible to non-specialists.
The Virgo gravitational wave observatory uses machine learning and deep learning at almost every stage of its data processing and analysis. In a recent review article, Elena Cuoco explains how machine learning is being used to track down sources of noise in the Virgo detectors, speed up simulations of the compact object mergers that generate gravitational waves, classify and characterize the gravitational wave signals that are detected and much, much more!
If you’re interested in the details of the Virgo analysis software, then you can visit this website to find out more about an Open Source analysis package that has been used to generate several exciting scientific results and search for new types of gravitational wave emitters like core-collapse supernovae!
You can watch a video about the use of machine learning by Virgo here.
Ground-based gamma-ray telescopes like the Cherenkov Telescope Array need to sift through millions of images captured by its telescopes every night, searching for elusive signatures of very high energy gamma rays among an almost overwhelming background produced by charged cosmic rays. Even once the gamma rays have been identified, determining their energy and where they came from isn’t as easy as you might think!
If your interested to learn about the ways that CTA researchers are using machine learning and deep learning to help find and characterize gamma-rays in their data then the CTA team has published several journal articles that you can read to find out more:
If you’re interested in the technical details then you can take a look at some of the Open Source analysis software that has been developed by CTA researchers.
Take a look at the web pages for GammaLearn: Deep Learning for Imaging Cherenkov Telescopes Data Analysis, or explore the source code for GammaLearn and CTLearn.
Particle physicists working with the Large Hadron Collider at CERN are using machine learning for a huge number of data processing and analysis tasks. In fact, the rate at which new models and technologies are being adopted and applied is so high that the high energy physics community has produced a "Living Review" that researchers can continuously update with new articles and reviews as they are published. You can also find lots of helpful links to lectures, tutorials and workshops on GitHub.
Optical and near-infrared astronomers working with data from the European Southern Observatory’s telescopes are developing ingenious techniques that use deep learning to categorise the spectra of astrophysical objects and distinguish different objects that overlap in crowded fields of view. Please find more details in this journal article.
In March 2021, ESCAPE partners organised a workshop on innovative workflows in astroparticle physics. Take a look at the presentations from Wednesday March 10th, which focussed on machine learning and deep learning methods.