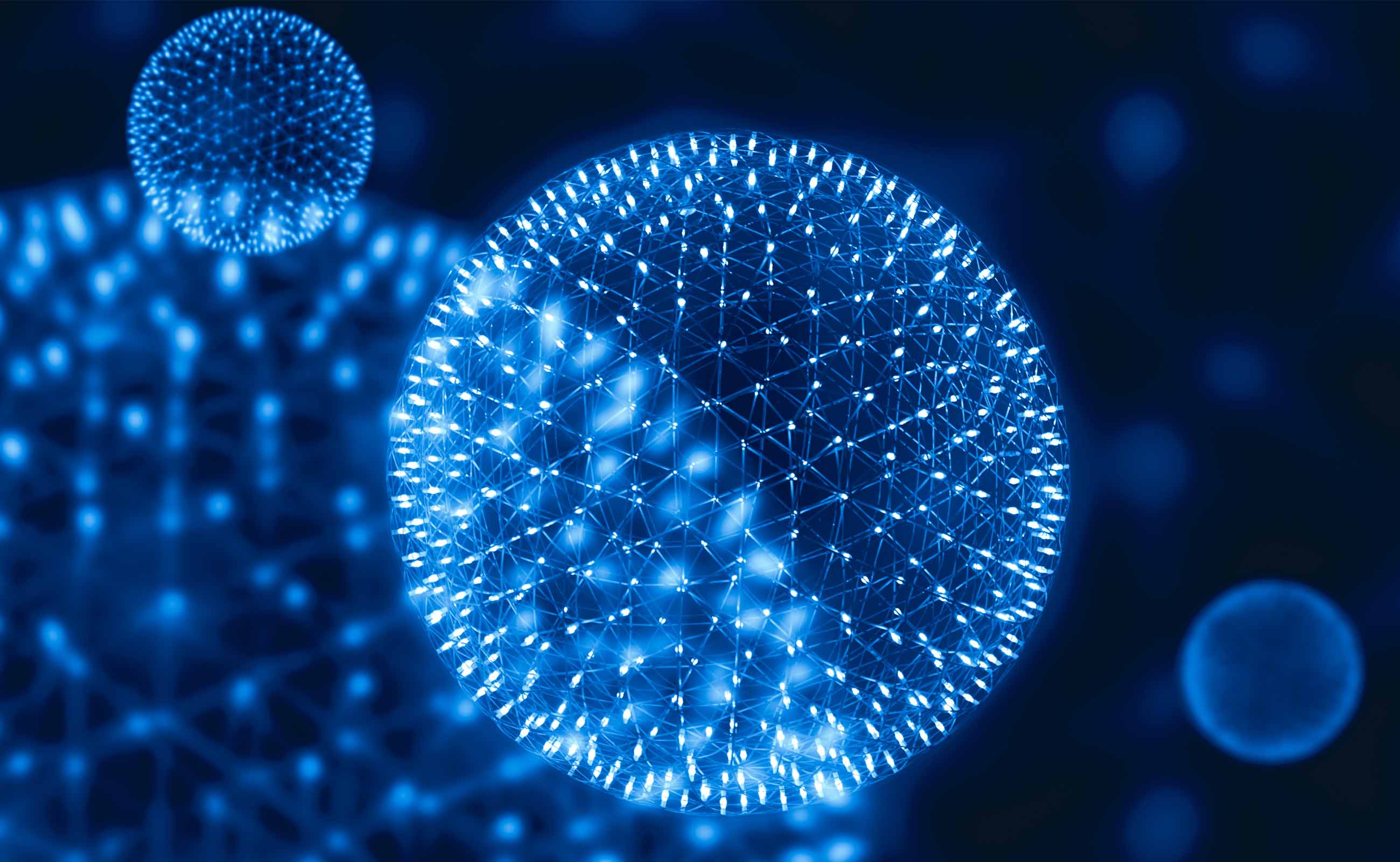
ESCAPE has successfully tested new machine learning prototypes that have improved search capabilities in some European Strategic Forum on Research Infrastructures (ESFRI) archives. By applying Artificial Intelligence (AI) deep learning techniques, ESCAPE proved the viability of enabling archival searches based on data similarity. Researchers in ESCAPE were able to upload data they were interested and, in return, the system provided matches based on similarities in the data itself, as opposed to the traditional metadata description. With this, researchers have more flexibility to retain the model to their specific needs, despite having some limitations regarding the precision of data items representation.
The work carried out within ESCAPE has the potential to enhance the discoverability of data in ESFRI science archives, thus prospectively enabling deeper levels of Open Science.
ESCAPE adopted a novel AI explorative approach, rather than supervised learning methods, to improve data discovery in broad variety of archive assets, considering the multitude of use-cases that scientists are involved in.
With millions of data files being made available to the science community, it is crucial for archives’ success to provide advanced query capabilities to guide the researchers in identifying data which are of interest to them.
ESCAPE is exploring novel AI techniques to increase search capabilities of ESFRI archives by exploiting the full content and scope of the data itself. The techniques were developed and tested by the ESCAPE partners European Southern Observatory (ESO), Heidelberg Institute for Theoretical Studies (HITS) and Centre de Données astronomiques de Strasbourg (CDS).
Usually in AI, supervised learning methods are typically trained to solve specific tasks like classifying astrophysical sources or extracting properties via a regression approach, both of which would be well suited to the current schema of database access. Even though supervised learning has often shown excellent performance, it is limited by its need for high quality-labelled training data, as well as the limitation that each model is trained to only solve a very specific task.
Lowering the barriers to data access is an integral part of the ambitions of Open Science initiatives such as European Open Science Cloud (EOSC), to broaden the use of the data itself. To help researchers in pursuing they scientific goal, it is crucial that they can find and use data, without having a specific knowledge of the source.